Vital’s 5 Guidelines for Using AI in Healthcare
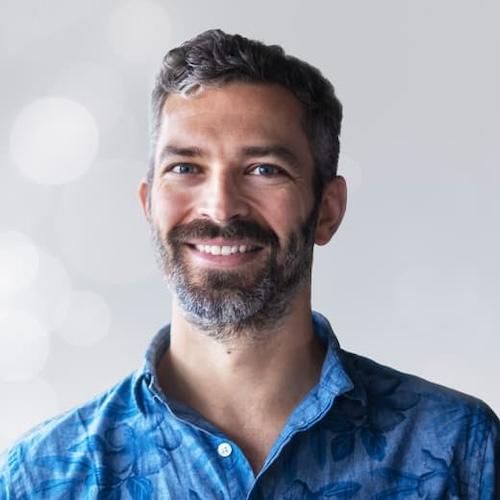
A few weeks ago, I had the honor of speaking at the Ai4 conference in Las Vegas. During my presentation Algebra, Cats, and Medical Jargon, I talked about how Vital’s artificial intelligence (AI) is being used to convert physician notes into more patient-friendly language.
Vital has dozens of AI models, ranging from LLMs to transform discharge documents into a patient checklist, to convolutional neural networks for early sepsis detection. We’re not alone. With a near-endless number of AI applications launching at the moment, it’s important for us to set some guidelines on what AI in healthcare should look like.
As the market for intelligent algorithms in healthcare grows from $14B in 2022 to $164B in 2029, we hope others might use this framework as well.
#1: AI should be for people, not profits
The most “successful” AI in healthcare today are tools for “ensuring all services are billed appropriately.” That’s an industry euphemism for “up-coding,” the practice of scraping every last insurance dollar out of a medical encounter. Only problem is, it doesn’t do a damn thing for patient health. It’s after-the-fact, when the visit is already over.
Insurance companies know about up-coding and aggressively combat it with their own AI. It’s an AI-arms race that does nothing to improve actual health, and everything to increase US health costs. Turns out the death of us due to AI is not SkyNet, but AI-powered bureaucracy.
First and foremost, ask yourself: will this AI help patient safety, understanding, communication, accessibility, or help save the patient time or money? Will it make patient’s lives easier? That’s your true north filter.
We try to do this ourselves. That Doctor-to-Patient Translator I mentioned at Ai4? It’s free worldwide, for patients and families to use. Need help understanding your parent’s diagnosis (maybe they are too old to remember what the doctor said they should do)? Use the translator. Trouble understanding bizarre healthcare billing codes or lab results? Use the translator.
We made it free for everyone because, well, we already built it for use inside the products we do sell. Why not make it free to help even more people understand their own healthcare?
Who else puts the patient first? Viz.ai has dozens of AI models to help radiologists detect neuro, vascular and cardiology events with AI-precision. That’s pro-patient-safety. Ribbon Health, Care Journey, and Turquoise Health offer cost, quality, and price transparency that’s decidedly pro-consumer.
#2: AI should help clinicians, not replace them
Software should automate what’s tedious, like surveying, or picking video education, or routing service requests to other departments so the nurse doesn’t have to fetch water, phone chargers, customize your food order, or do the million other things that frankly cause nurse burn-out.
But AI can’t and shouldn’t replace nurses and other health care professionals. Most sepsis algorithms, notoriously EPIC’s beleaguered system, look at numerical data like vital signs, or at categorical data like a drop-down chief complaint.
At Vital, we’re working on more nuanced, accurate sepsis modeling that factors in free text observations from nurses like, “patient feels ‘foggy’,” or “their skin looks a little green.” These are intuitive observations only humans can make — a combination of feeling and experience that something is off. But once written, they can be used to train a sepsis model that works at the time of triage, usually 8 to 20 minutes after patient arrival.
Our algorithm is nothing without these human observations.
#3: AI should be explainable, with no black boxes
Most of healthcare is stuck in decision trees. These are pre-AI. They are pre-machine learning. For example, the ESI (emergency severity index) algorithm is used to determine the acuity of an emergency patient, 1 = most urgent, to 5 = not urgent. But 68% of people get lumped in the middle as ESI 3. This is the “dunno” category — the doctor equivalent of a shrug. Having a super-majority of patients categorized the same way is not that useful.
Why do decision trees for ESI, fall risk, coma score, and hundreds of other medical decisions still exist in the age of AI? They are easy for humans to perform, easy to understand, and typically have a peer-reviewed paper showing that the decision tree is much better than guessing. That last point comes in handy in medical liability lawsuits when opposing counsel asks for a concrete reason why a decision was made.
For AI to have real adoption, it must have the same level of transparency. While the “weights” of each neuron in a neural-net are hard to rationalize, the decisions the system makes are not.
Let’s say we have an AI that takes “heart rate” and “blood pressure” as two inputs. Which is more important? You can “wiggle” each input, changing each by, say, 1% up-or-down, but hold all other inputs steady. This allows you, through the byzantine route of neural weights, to determine which input most affects the output. That’s your explainability.
We apply a similar algorithm across literally tens of thousands of inputs, including every single word in free-text doctors notes. In our sepsis algorithm this might look like “++fever++ began this afternoon, ++102.4++. Denies - - n/v/d - -”. Here, sepsis is correlated with “fever” and “102.4” but diminished by “denies n/v/d.”
This allows us to pull the most relevant bits from a 10-page medical note for human review. It’s a bit like a Google search “snippet” showing the matching bit in the suggested website.
#4: AI should be judged by “what’s better,” not “what’s perfect”
We had a panel of experienced physicians review around 2,000 imaging results from our Doctor-to-Patient Translator. Input data was geographically dispersed across the US, and included X-rays, MRIs, CT-Scan, and ultrasounds for patients of all ages.
This panel marked 99.4% of the translations as “safe” accurate summarizations of a typically much longer medical note. What about the 0.6% (roughly 1 in 200)? What went “wrong” there?
So far, we’ve never seen the system make negation errors: saying you have cancer when you don’t, or vice versa. We’ve never seen it mix up a medication instruction. But sometimes, when trying to whittle a complex 10-page report for a patient with many underlying conditions into three to four sentences, it misses something that doctors consider important to health. Should we put it out to patients? Is 95% acceptable? 99.4%? 99.99%? There are no official industry guidelines. Use your clinical review committee, do an IRB study, and monitor closely in the wild during a 3- to 12-month trial.
AI is never going to be perfect because it is statistical, and patients are unique. But perfection is not the right comparison. How well would a doctor do summarizing a note by hand? Our panelists often commented that our LLM found important things buried in the notes that they themselves had missed at first glance. In other words, AI is probably better than most physicians at summarizing long medical reports safely. And that’s the real comparison. Aim for better than today, not 100%.
#5: AI should be free of biased inputs
Whether in pain medication administration, vaginal-after-ceasaren birth decisions, or kidney transplants, there are unfortunate racial and ethnic biases in medicine. Omit these categories from your AI training data. Omit proxy values too, like zip-code, income, and language spoken at home. Unless it’s needed operationally for care coordination, omit housing status, and type of insurance from AI training as well, including from free-text notes that may have been cut-and-pasted by your EHR.
These are our internal guidelines at Vital. What did I miss? Drop me a line on LinkedIn.
Learn more
Find out how Vital’s AI-powered solutions can help you serve your patients. Request a demo today.